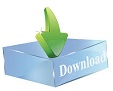


Phenomena such as Big Data, the Internet-of-Things, Closed-Loop Product Lifecycle Management, and the advances of Smart Factories tend to produce humanly unmanageable quantities of data. With the increasing amount of available data, computing power and network speed for a decreasing cost, the manufacturing industry is facing an unprecedented amount of data to process, understand and exploit. Our experimental protocol put forward the versatility of a regularizer that is easy to implement and to operate that we eventually recommend as the new baseline for future approaches to transfer learning relying on fine-tuning. These tests show systematic improvements compared to weight decay. We replicated experiments on three state-of-the-art approaches in image classification, image segmentation, and video analysis to compare the relative merits of regularizers. This paper demonstrates the versatility of this type of regularizer across transfer learning scenarios. Hence, regularizers promoting an explicit inductive bias towards the pre-trained model have been recently proposed. This choice conflicts with the motivation for fine-tuning, as starting from a pre-trained solution aims at taking advantage of the previously acquired knowledge. Fine-tuning requires some form of regularization, which is typically implemented by weight decay that drives the network parameters towards zero. This adjustment is nowadays routinely performed so as to benefit of the latest improvements of convolutional neural networks trained on large databases. Results on optical coherence tomography images of the retina demonstrate that the approach correctly identifies anomalous images, such as images containing retinal fluid or hyperreflective foci.įine-tuning pre-trained deep networks is a practical way of benefiting from the representation learned on a large database while having relatively few examples to train a model. Applied to new data, the model labels anomalies, and scores image patches indicating their fit into the learned distribution. We propose AnoGAN, a deep convolutional generative adversarial network to learn a manifold of normal anatomical variability, accompanying a novel anomaly scoring scheme based on the mapping from image space to a latent space. Here, we perform unsupervised learning to identify anomalies in imaging data as candidates for markers. High annotation effort and the limitation to a vocabulary of known markers limit the power of such approaches. Models are typically based on large amounts of data with annotated examples of known markers aiming at automating detection. Obtaining models that capture imaging markers relevant for disease progression and treatment monitoring is challenging. We study GAN performances to retrieve geometrical parameterization of surfaces. The DMD is a geometrical parameterization technique using a modal space projection to geometrically describe surfaces. Moreover, we introduce the innovative use of Discrete Modal Decomposition (DMD) to analyze network predictions. We firstly study prediction performances using different image similarity comparison algorithms. Our dataset is really small and the GAN learns to translate thermography to geometry. In this paper, we use recent success of Generative Adversarial Networks (GAN) with the pix2pix network architecture to predict the final part geometry, using only hot parts thermographic images, measured right after production. Thus, the final part geometry must be predicted from measurements on hot parts. To guarantee the product’s quality, it is necessary to adjust the process settings in a closed loop.Those adjustments cannot rely on the final quality because a part takes days to be geometrically stable. Geometrical and appearance quality requirements set the limits of the current industrial performance in injection molding.
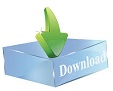